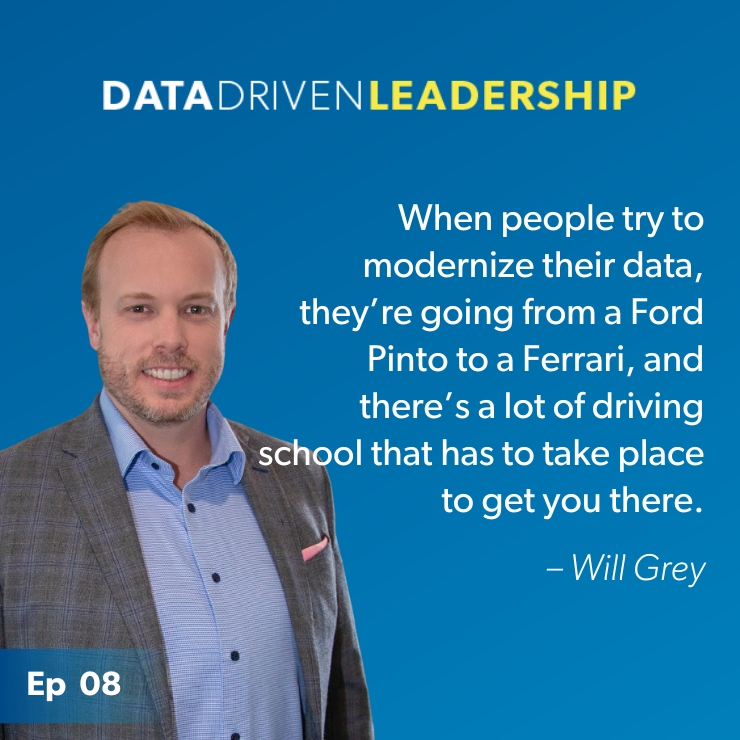
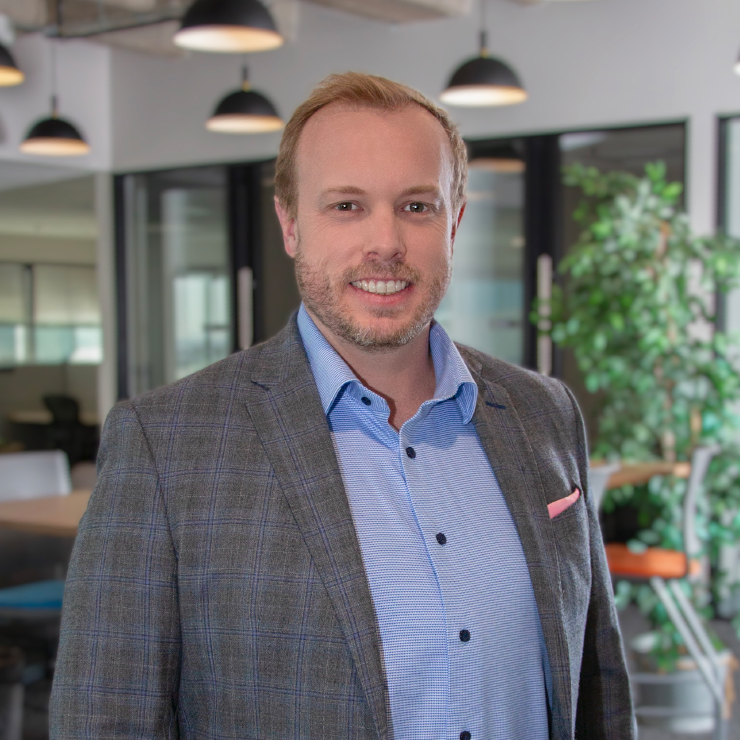
Transcript
Jess Carter: The power of data is undeniable, and unharnessed, it's nothing but chaos.
Speaker 2: The amount of data was crazy.
Speaker 3: Can I trust it?
Speaker 4: You will waste money.
Speaker 5: Held together with duct tape.
Speaker 6: Doomed to failure.
Jess Carter: This season we're solving problems in real time to reveal the art of the possible, making data your ally, using it to lead with confidence and clarity, helping communities and people thrive. This is Data Driven Leadership, a show by Resultant.
I'm your host Jess Carter, and on this episode of Data Driven Leadership, we're diving into modern data warehouses. Specifically, we're going to look at the issue of single sources of truth in your data and ultimately, how do you get there. We're going to get this answer in the first few minutes of the show with Will and afterwards, we'll have two experts who take a real life example and break it down for us.
To help me solution on the spot is Will Grey, VP of Data Services at Resultant. Welcome to the show, Will. We're so glad you're here.
Will Grey: It's good to be here, Jess. Thank you for having me.
Jess Carter: Yeah, and Will, for those who don't know you, can you just give us a quick insight about why Will and why data warehouses? Where'd you kind of land in this space? How'd you get here?
Will Grey: That's an interesting question and I'm not actually sure how I landed here either. I started off in store operations for both Walmart and Target and then somehow stumbled into analytics. And my previous organization was going through a data warehouse project, and it was probably one of those that was on the Gartner statistics but I'm on the positive side of it.
And so I got to see a lot of what not to do and then I latched onto a few key technologies in my career, and just went deep dive and just fell in love with the space and how you get the business to really adopt analytics and become data driven. And that led into my career as a consultant. I have the pleasure of serving a team here as the VP of Data Services.
Jess Carter: So if I hear you right, your career really started in the business itself and you needed data, and then you kind of fell into that data component for a new career move, right?
Will Grey: Exactly.
Jess Carter: That's awesome. Very cool. Well then you'll be the perfect person for our solution on the spot today. So are you good if we head into our solution on the spot segment?
Will Grey: I would love that.
Jess Carter: Here we go. You and I are getting called into a potential client's office. It's a CIO. It's somebody that's been friends with you for a while and their new CIO to an organization that's had a warehouse for a while. But as they get started into their new gig, they're realizing that leaders of different departments in the business are coming to them with data that's supposed to be operational insights, but it's conflicting.
And the more they dig into the warehouse, they're realizing it has major issues that needs resolution and they're trying to discern do they tear it down and build from scratch? Do they try and fix it? They weren't looking at a project or a strategic plan where they wanted to go build a data warehouse, they just wanted the operational insights. So a little overwhelmed trying to figure out what to do, where do you begin?
Will Grey: Oftentimes, we begin just with a conversation. It's a two or three-hour workshop that exists on a whiteboard, what I have behind me. What we often see is there's tension between business and IT. So everybody likes to point the finger at the other party of why do numbers not match, why can't we get accurate reporting or why aren't we getting our forecast correct?
And what we end up seeing is in most organizations there's not a source of truth or a single source of truth. It's sources of truth. Finance has its source of truth. Commission, sales has its source of truth. IT is trying to replicate and reconcile both of those together. And oftentimes you see that in a data warehouse project.
And what we often see as well and when we're assessing a current standup is the data warehouse was built six years ago. Everybody felt really good about it about six years ago. And then there was no maintenance done to it or the very minimal. We still walk in and we see 2008 or two SQL Server 2012 more often and that's it. They're on-prem otherwise. They're in AWS and early version in Redshift or something like that.
But nobody's really leveraging that, they're taxed out. And what I often find more than anything as consultants, our job is to might be more of a marriage counselor and to try to break down and figure out how do we make this work? We signed up for this, how do we move you to something that's more modern and that truly solves the problem.
Jess Carter: And we should probably back up for a moment here for the dynamic too of, I haven't even really said, what is a data warehouse? We should probably just cover that too. When somebody says, "Hey, I've heard about these things. What are they? Can you give a quick elevator pitch of what is a data warehouse and when might you want or need one?
Will Grey: My definition would probably not match what's in the textbooks, but what I would say is a data warehouse is something that collects and centralizes your data and maps out according to how your business flows. And so it allows your data to flow like the red map out, how your revenue and how your business makes money.
And so it should be a reflection of that. And that's oftentimes where we see IT departments get in trouble is they build their data warehouses mapping out their source systems, and they don't map out the ontology, the overall working scheme of the business. So that's kind of the differentiator, I believe, that why we outperform and don't see the failure rates of the data warehouse project any organizations do.
Jess Carter: When I play through some of my experiences working on data warehouses too, there are some behavioral issues that are really interesting too around data warehouses. So I've noticed leveraging the data as a weapon, so how do I make my team look like they're doing really, really good. And I can go build a report that says exactly what I want it to, but it might be contrary to how somebody was using that same source system data to make their department look really, really good.
And so magic word of a marriage counselor, there's this like, "Hey guys, it's not just about replicating the data that was in your source system. It's also about agreeing and aligning across the org on what metrics and data we're going to leverage to drive the business, and making sure that we're analyzing it appropriately as a signal for the business, not as a performance eval tool for your leader or manager."
Will Grey: It's spot on. You made me think back to early in my career in analytics where I had somebody come in and say, "No, I need you to come back with different numbers. You're not analyzing correct." I'm like, "No, it's correct. This is what sales are. Sorry, it doesn't need the story. Here's how I would pitch it."
We often see the conflict happen specifically between finance and then kind of your sales revenue generation department specifically because one is trying to say, "No, this is what's happening and this is our definition of what you do." And sales is like, "No, this is what we generated. This is what we're going to pay commissions on. This is our definition."
And oftentimes at the base layer it's the same, but it's about what you include or don't include in the different filters. And so you say one number but exclusions on what might be included or not is just a difference in definition. And that's really risk in building a data warehouses and when we're scoping, is how long does it take for us to figure out what is that real definition? And that's what causes a project to either go long or finish up really quickly.
Jess Carter: And even does a client come to you and really know immediately what they do want or does it elaborate? Because I think some of the other experience I've had is as we start to build momentum, so if we're working with this client, we discern that their hardware and software is out of date. Everything is out of date, we need to just of rebuild.
As we get those filters layered in, we get the sources plugged in, they start to get this momentum of like, whoa, there's value here. But then you got to manage and govern it because then people want to build their own filters.
And so there's this organizational purview of how are we going to use this tool to drive meaningful decisions? And you can get to those performance metrics for your commissions and you can get to how is everybody behaving, but you also need to have those signals about how the business is performing, where the story tells itself. You don't try and fit the data to the story you're telling. Is that right? I feel like that's what I'm hearing you say.
Will Grey: It's layers. What I have is kind of my core layer. If you think about transactions or how our monetary system works, I have a dollar. That's the base of it. I'm going to hand you a dollar. Now that happens, there's another layer that's credit cards and so how does that transact or reconcile? And then on top of that, there's more layers.
And so a data warehouse is going to be the same. I have my core foundation principle that maps out the business and that's the data warehouse. And then I might have different analytical access points to the data that allow you to tap in.
And so if somebody wants to bring a Tableau or somebody wants to bring a Power BI or whatever the next technology stacks are, data science or Spark, to be able to drill in deeper into your understanding of machine learning, you can do that. And it's what layer do you trust and which layer do you allow for more of that little bit of freedom?
Jess Carter: So there's room to play, we're not saying you have to lock the thing down and only give keys out to three people. We're saying let's agree on what we're going to leverage to make data driven decisions for the organization, and then let's have some exploratory room to play where we can analyze the data and figure out if there's new insights that we can draw into how we manage the business.
Will Grey: Amen. Data governance is supposed to be an enabler. It's supposed to be, hey, here's the rails that keeps us out trouble and here's how we're going to know that we're going to be okay, and here's the paths not to cross. But it's not supposed to be ... Most people when they implement data governance, it's a scary thing and we're going to shut down access because we don't really know what to expect.
So it's the fear of the unknown. When you have somebody who's running data governance programs, who understand what are the things that are unknown the most but are known by us, then it becomes an enabler. It becomes that slingshot for your organization that says, "How do we get data out into the organization that people can trust and do things with?"
Jess Carter: So if we follow the story through, so this guy or gal we're working with says, "Hey, here's what they laid it out for us. We see the software they're using. We understand the analytics they're trying to overlay on that data to make decisions. We see how complex or simple it is, either way, you're going to start to understand it and propose changes they need to make whether it's a new filter or a completely new solution."
And maybe that's the question too is I think some people want to know or maybe this client might ask, "When will this ever be done?" There's sort of this vibe that data warehouses get of like it's this continual improvement. And so if this is somebody who was never supposed to be in the business of a data warehouse this year and now they're finding themselves in the middle of trying to scrape together a budget for a data warehouse solution, how much does it cost? How long does it take? When are we done? How do you tackle any of that?
Will Grey: I usually answer it head on. Great data warehouse is never going to be done, but it's going to be built in a way that is scalable and that you can know that there's always some iteration or improvement. But you have a workflow that maps it in. Because a growing organization is going to be one that is always finding new data sets, it's figuring out how to monetize their data, it's figuring out how to interact with new vendors and how think differently.
And that happens on the edge. So, that happens in your marketing department. That happens in your sales or revenue generation market. That happens through analytics and [inaudible 00:11:33] partnerships. But I think differently and say, "Oh I could tap into that data source or I can do this."
And then it's about asking the right questions. Well, why? And it's oftentimes you have to get to the five layers of why beneath so much so that a lot of IT departments make the mistake of saying no, instead saying, "Not now. Let's get the plan together so we feel really good about it."
Jess Carter: So the right people involved in the engagement matters, the right information and decisions. Learning those quickly matter. And then I don't know, does it take a quarter? On average, if you're looking at an enterprise company, maybe like mid-level enterprise company, 500 people, I don't even know if that's helpful to you when you discern the length of this. But how do you discern for this guy or gal? Is this going to take 16 weeks, 6 weeks, 6 hours?
Will Grey: That's usually after the first conversations where you're going to know what the length is. Because we've built large organizations data warehouses in three months and we've built them in 12 months. But it's how easy it is to extract the business roles and how much digging do you have to get to do that? How complicated are the source systems and then what stack do they use?
Most people are trying to modernize and so they're going from a Ford Pinto to a Ferrari. There's a lot of driving school that has to take place between to get you there so you feel comfortable on the track with that. So I think the same with a data warehouse and something that's skipped over quite a bit is the change of management process that goes along with it.
And so making sure that the rest of the organization knows how to tap into it and really leverage the insight coming from it and they can trust it.
Jess Carter: Thanks for your time, Will. The conversation we just had is a perfect intro for the longer conversation that you're going to listen to now. The two experts you're going to hear from are Dave Haas and Michael Tantrum, two business executives at Resultant who are amazing and have tons of experience in data warehouses.
Insights you're going to hear in this next conversation include quick tips on how to begin a project like this, what are some common mistakes to try to avoid, and what to do once you release your first iteration?
Dave Haas: I think the best place for us to start is around the definition. Could you perhaps help us define exactly what a modern data warehouse is for our audience?
Michael Tantrum: Yeah, sure. Most of you on the call will be familiar with the concepts of data warehousing. And the question is how does a traditional data warehouse differ from a modern data warehouse? And there's not really one core definition, but there are themes that tend to occur in the modern data warehouse space.
The first most logical one is that traditional warehouses have been on-premise. So they've been your classic, your SQL servers, your appliances like your Teradatas, [inaudible 00:14:22], et cetera. The modern data warehouse almost overwhelmingly is going to the cloud.
The next thing that seems to be thematic is the frequency of refresh. So traditionally, we used to get away with an overnight, sometimes weekly refresh of data. The modern data warehouse is moving towards more frequent intraday and even towards real-time refreshers. Not always, not all data requires this and not all organizations desire this.
Another theme is data sources. So traditionally, data sources were relatively straightforward. Originally we worked with files and relational data sources. The modern data warehouse has a lot more types of sources. So web APIs, we still got the traditional but we've got things like web APIs. We have IoT. We have SaaS services, a lot more variety of sources, sensor data and things like that.
Another theme that we see, the traditional warehouse often was very manual in the way it was developed. So if we look at the development style, it was quite manual. A modern data warehouse should contain a reasonably high degree of automation. And automation has many faces. It could be design automation, it could be development automation, it could be documentation, it could be deployment automation, it could be CI/CD, it could be data governance automation, testing automation. But automation should form a core part of a modern data warehouse.
Traditionally, of course, data warehouses were just your classic databases. But the modern data warehouse and landscape also includes elements of data lake. And you get all these various hybrid models. You'll start to hear of things like lakehouse and data shorehouse and things like that, so a mixing of the concept of a data lake. We've had data lakes around for a while, but the role was always as a repository of data, not necessarily forming part of your core analytic landscape. So that's one of the things there.
The final thing I'd mentioned is data governance. In a traditional data warehouse space, data governance was almost an afterthought. It was a nice to have. It was if I actually managed to get everything else done, then I would think about data governance. A modern data warehouse data governance is being built in from the beginning by design. And so it's quite a different approach.
People are now demanding to be able to say, "What is my data? Where does it come from? How did it get there? Can I trust it? Is it of good quality and such?" So those are probably the main identifying features, I would say, of a modern data warehouse.
Dave Haas: Excellent start. It gives us good framework to build on. So let's just dive right into it from there, Michael, let's talk about why you actually need a modern data warehouse.
Michael Tantrum: So the need for analytics is only going one way. And those of you who do this for a job, you know what your entry is like. You know the request from your users for more variety of data, for more types of analyses, for frequency, for complexity, that's only going one way.
And so we need, as IT professionals, as data engineering professionals, we need to be able to adapt. And it's always been our problem. We've struggled to adapt in the traditional sense. We have to be able to adapt much, much faster in the modern world.
So the increase in data, increase in types of data. The other thing is cost. So the cost of infrastructure in the cloud makes a lot of the traditional approaches very expensive. And as your existing data warehouses reach end of life, so your servers, your software and database platform licenses start to come up for renewal, it's a good opportunity to say, "Can we make a step change in the cost of our data warehousing?"
So the cost of cloud storage is dropped through the floor. The cost of infrastructure of managing data centers is really cheap. And it becomes a question from a personnel point of view, from a cost, from a security, from a hardware point of view, does it make sense to do it on-premise anymore? And so the cloud is driving a huge opportunity for people to consider it's now the time to rework and modernize my warehouse.
The final thing is your end users are getting increasingly sophisticated. In the past, they would've been okay with the dashboard or maybe some reports. Now, they're wanting integrated analytics. They're wanting analytics with workflows that change the way frontline people operate the business. To adapt, we've got to go to new ideas.
Dave Haas: Michael, I see this frequently and it's interesting, we kind of tend to see four groupings of folks in their journey around this modern data warehouse operation. And I think if we draw kind of a sector, it'd be kind of interesting to hear you talk a little bit about where you see people sitting. If we on one access perhaps, let's call this value of your data. And on this access, let's call it access to data. It'd be interesting to kind of get your perspective on each of these quadrants.
Michael, at the bottom right here, we have an area where you essentially are in a spot where you're not getting any value from your data today. You have a very difficult time accessing your data. What might we call that?
Michael Tantrum: I think most organizations, you'd probably call this something like the hurt zone. And this is where your users are, because they can't get at data, they're running their analytics off spreadsheets. They're managing by instinct, they're not using data-driven decision making, they're not leveraging the data that they have. And this is not a good place to be. They're not getting value from the data in the organization.
Dave Haas: Now, how about this top left zone, Michael, where people are accessing their data but unfortunately, they haven't modernized their data warehouse yet and they're not really seeing any value?
Michael Tantrum: So we've pushed some data out to our users in some form, maybe through Tableau or static reports or even nice websites, but they're not getting value from it. So what's happening here? We've got low engagement with our users. Probably they haven't had a lot of input into what we've built for them. They're not getting business value from it. And there's probably a lot of questions around what did we spend this money on?
And so your issues here to try and move to a better place, you've got to say, "How can we increase the engagement with our users and answer the question why don't they have value? Have we asked and answered the wrong question? Have we built a laboratory experiment rather than something the business actually needs?"
If I'm going to give this a name, let's call us the access zone. I've got access to data, but I'm not really doing much getting any value from it.
Dave Haas: Excellent. So Michael, let's go towards the bottom right zone. Again, I think this is kind of a yellow zone, well for lack of better words. But what might you call this zone and what are some of the characteristics of the folks that-
Michael Tantrum: So this is where the business users, they're getting a lot of value from the data, but they just can't get at it or can't get at it well. So let's call this the desperation zone. And so here, what you guys will see is your users spending a lot of time assembling and manipulating data. Now, we don't want them to do that. We want them to focus on making decisions and using data to drive the business. We don't want them having to assemble and manipulate it.
And so you will see people trying to use desktop blending tools like Alteryx. If they're a bit smart, they'll be trying to use their own copies of BI tools, they'll be using spreadsheets. They may even try and stand up their own sort of data marts in an attempt to get somewhere. But this is not what we want business users doing. We want business users running the business and letting the data professionals focus on the data side.
So again, this is not a great space to be. We want to increase access to data. This is where we want to be providing systematic automated approaches to providing user access.
Dave Haas: All right, Michael, we've got a zone here to the top right. I'm going to make this a green zone. It's a pretty good place to be. Let's talk about what some of the characteristics are and what you might call this zone.
Michael Tantrum: Yeah, good access to data. So we are supplying repeatable, reliable, structured data to users to make good decisions on. They can rely on it. We might call this the strategic zone. And this is probably where we all aspire to be. We are getting value and people feel like they can make good business decisions based from this.
And you think, "Okay, I'm in the strategic zone, I'm good." High five, modern data warehousing. Maybe I'm tweaking up some of the tooling underneath, but can I get better? What can we describe that as? Can we create maybe a ... Yeah, let's create a pocket in there. Let's call this the elite zone. And what does the elite zone look like? This is secure data, it's governed data. It's highly reliable, well populated. You're adding new data sources at the speed that business want to consume it. This is where we want to aspire to.
And what I'd actually encourage you all to think about here is think about these axis and maybe scale them from 1 to 10 and grade yourself. How do you think your business users score on feeling about their access to data and the same with the value of data? Work out what zone you're in and maybe consider where you'd like to go, and we can talk about how you move that towards the green zone and then ultimately to the elite zone.
Dave Haas: Excellent. This is just a great kind of visualization, a way to kind of figure out, all right, as I'm planning my modernization project, my data transformation project, having a sense of where you are and how you're going to get there basically is so important, just to start off. But from there, let's transition a little bit and let's talk now about some of the common mistakes that you see folks making with this journey.
And what I'm going to do here, Michael, is I'm going to draw, call this my iceberg drawing. And if you could kind of talk about as the tip of the iceberg, some of the real common mistakes that people essentially make that are pretty easy to spot. And then perhaps underneath the water, let's talk about the ones that are not so easy to spot.
Michael Tantrum: Exactly. Yeah. And like in iceberg, what seems obvious, this seems to be the problem is not usually the problem. So if I'm looking the part of the iceberg above the waterline, so to speak, the things that you would be noticing here is that you are struggling to deliver your projects on time. You've got requirements which seem to be constantly changing, and you're chasing your tail trying to stay on top of it. You're doing a lot of rework.
It just feels like you're forever doing re-engineering, just trying to get things right. You're struggling with unforeseen data quality issues and they just keep biting you. And your end users are not engaged and can't be satisfied. And so often, those are just the symptoms. But we look at them, we say, "We've got to solve these." But the reality is if I drop below the waterline, what I'm seeing is a different thing. What were the mistakes that I made that caused those things to be the case?
Well, first I think the common one is you just didn't engage people who've done it before. So you're trying to build it yourself for the first time. You took a build versus buy approach. Now that also relates to people. You have the option to recruit and hire expert hires who can help, or you can use consulting firms. But the first problem was you didn't get people who've done it before and you're trying to do it yourself.
Another thing that could have happened is that you haven't put your best business analysts or your best subject matter experts onto the projects. Now these people fight joining data projects usually because they have a day job. And so we're asking them to give up some of their day job time to help us work on the project. And the other critical thing with these people is they must be empowered to make decisions on behalf of their business units about design.
So if you have a question, "I have this data. It doesn't look quite right or how do I want it grouped or categorized or this business rule calculated?" I need someone who can say, "Do it like this," and not have to go back and consult a committee. If you do that, your project is doomed. So they've got to be empowered, smart people.
Other things you'll see a lack of attention to things like architectural standards. We talked about the standards up front, design standards, development standards, documentation standards, deployment standards, operational standards, lack of attention to standards at the beginning.
Also, testing, a lack of consideration of data testing in QA as a core part of your process. Probably you may not have made good choices around tooling. It's easy to get seduced by a tool without considering the wider context of what are the modern tools and how do they bring automation to the party.
And I think the final thing which bites people is not taking an agile iterative project approach. But this is still not the core of the problem. Can you draw me the bottom weighty part of this and make it a red or something? We'll call this the kill area. This is where the things that really kill projects. And if they're not considered properly, everything above it is doomed to failure. And the key here is strong executive sponsorship and a product champion.
This is going to be as high up in the organization as possible. This has to be someone who will marshal resources, will defend your project at the board level, will make sure that the goals and strategies of the company are aligned to the delivery. And so a big failure here is a lack of aligning to say, "Why am I building my warehouse? What is the business purpose? What do I need to run the business? And is my data warehouse aligned with those company objectives?"
Another problem is expectations. It's a little bit like Goldilocks, how long is this data warehouse going to project to be? You can set your expectations too short and you can set them too long. That's why I call it the Goldilocks problem. You got to set it just right. If you don't get good expectations set, people get frustrated about cost overruns or delivery timelines.
You haven't considered an adoption strategy. The saying, build it and they will come, all too often we build it without considering whether people will come. And so working with your end users about adoption is a critical component because otherwise, if you build it and they don't use it, it's a white elephant. You got to build up their confidence that what they have is right.
The other thing I think is that people forget that this data warehouse you build is a living organism. It doesn't stop. There is no done. There is no end. And what that means is as you build, if people are using it, they're going to be asking for new subject areas, changes in business rules, additions, more frequent data.
And that's a good sign because it tells you that people are using your warehouse. What it also means is you have become the victim of your own success. And so don't consider, I build it then I'm done. You've got a plan for the life of this living beast, and you've got to give it care and feeding throughout its life as it grows.
Dave Haas: Thank you, Michael. Do want to touch on iterative development for a few minutes? Could you talk to us about what that looks like and what people are doing today to get to where they need to be?
Michael Tantrum: Yeah, very good point. So the classic mistake people make here is that they treat this like any other project. And so they treat it like a staircase. So maybe draw me a staircase there and I start on the bottom step and I say, "Right, what do I need to do? I need to gather some requirements." So I gather the requirements, then I need to ... Haven't got the requirements, I come up with my architecture and my approach and set up my environment.
And then I do my build and then I push it out for deployment. That sounds textbook development, project management. But here's the problem. You're dealing with analytics. You're dealing with people who cannot articulate good requirements. They can tell you the first problem they want to solve. They cannot tell you the six questions that are going to come out of their first answer.
And so if you take this approach, firstly, they're not going to be able to give you good requirements. But if you insist and you say, "I can't build it if you don't tell me what you want." And so eventually, they'll give you something and then you're going to deploy it, you're going to put it into a user acceptance testing environment. The users are going to look at it and they're going to say, "Ah, now that I see it, can I have some changes?"
And you're going to have to go all the way down the staircase, update requirements, new requirements, changing requirements, rebuild, and you're running up and down the staircase. And it becomes exhausting because there's just so many moving pieces to have to work with. And that's a hard way of doing a data warehouse. It's the right way to build an operational system or maybe a website or something like that. But the right approach to dealing with data warehousing is an iterative approach.
And so with an iterative approach, you start with an idea. A user will come to me and say, "I need something like this." And often, and people laugh at me for this, I will give them a whiteboard marker and say, "Draw what you think you need." So I'll take that idea that they've drawn and I'm going to build a prototype. So the first step is give me a little circle, we'll call this an idea, and then I'm going to build a prototype.
Now, once I've got that prototype and this prototype, if it takes me more than a few days to build, then it's not a prototype. I'm going to put this in front of my user, I'm going to call them back into the room, throw it up on the big screen, or throw it on the Zoom screen in a COVID world. And I'm going to iterate that with them. I'm going to sit them down beside me, maybe figuratively in a COVID world, and I'm going to iterate.
And I'm going to say," Okay, what do you see there? What needs changes?" And they're going to refine their requirements as I redo the building. And eventually they're going to say, "What you have on the screen right there, that's what I want." And we'll say, "Okay, now I'm going to deploy that." And so step number four is into a deploy. And then we go back into the idea cycle.
And this concept of what I would call conference room development is the only way to help my users refine their requirements, but in a way that I'm able to manage my development process. So prototype iterate is a highly effective way of developing data warehouses.
I will say to make the iteration effective, you have to have the right tooling. And there are a variety of modern automation tools out there with code automation, modeling automation and things like that. We've got lots of ideas. You guys may be using some of them, depending on whether you're building data vaults or more traditional Kimball warehouses. There's all sorts of options there. But you've got to consider automation tools because otherwise your iteration cycles take too long.
Jess Carter: Thanks for listening. I'm your host Jess Carter, and don't forget to subscribe to our Data Driven Leadership wherever you get your podcasts. And rate and review us, letting us know how these conversations are shaping your business. We can't wait for you to join us on the next episode.
Insights delivered to your inbox