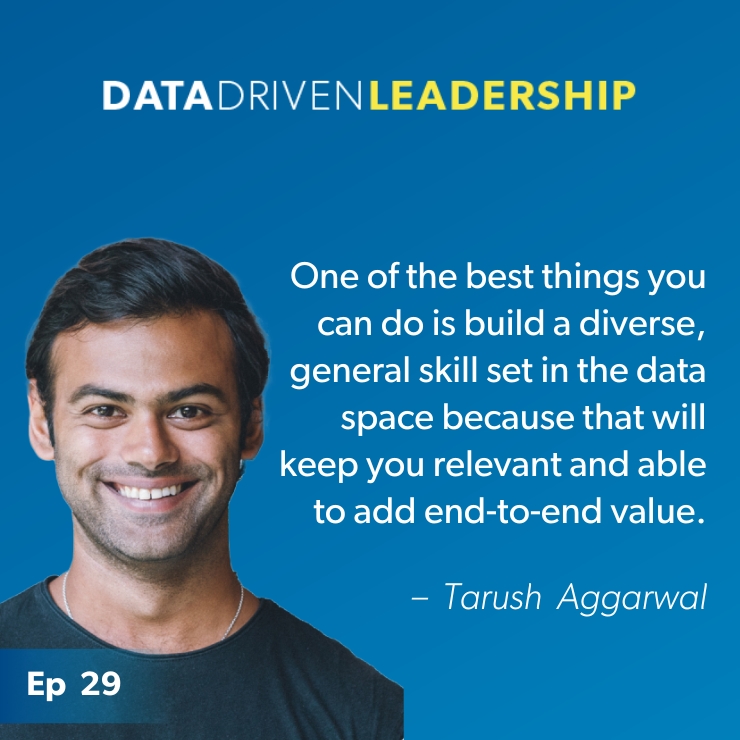
Is the Rise of the Data Generalist the Next Step in the Evolution of Data Strategy?
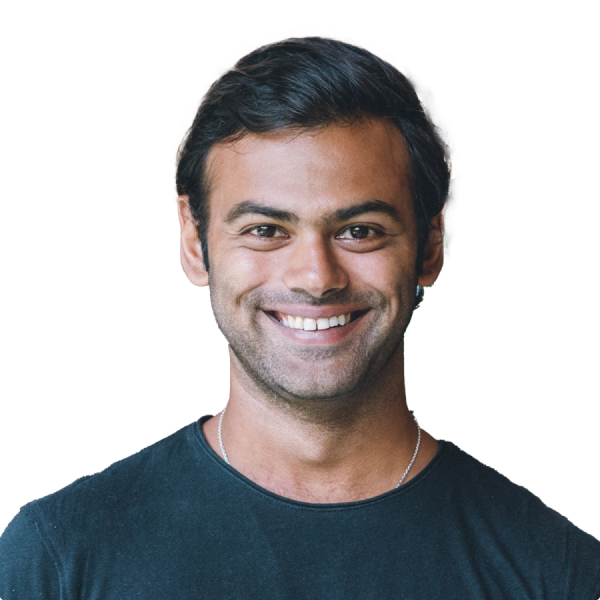
Transcript
Jess Carter [00:00:01]: The power of data is undeniable and unharnessed. It's nothing but chaos.
Speaker 1[00:00:06]: The amount of data, it was crazy.
Speaker 2 [00:00:08]: Can I trust it?
Speaker 3 [00:00:09]: You will waste money.
Speaker 4 [00:00:11]: Held together with duct tape.
Speaker 5 [00:00:12] Doomed to failure.
Jess Carter [00:00:13]: This season, we're solving problems in real-time to reveal the art of the possible. Making data your ally, using it to lead with confidence and clarity, helping communities and people thrive. This is Data Driven Leadership, a show by Resultant.
Hey, guys. Jess here. I just am excited for you to listen to this conversation about Tarush Aggarwal and his company, 5X. I think what's really interesting and what I appreciate is he's really in tune with his life. I think he had kind of this interesting 2020 experience trapped in Bali, and he'll explain some of that in the episode.
Jess Carter [00:00:55]: But it was right when he was sort of rethinking a bunch about his career. And I think what comes out is this kind of whole person that is very good at what he does but is also very aware of kind of who he wants to be and tries to stay true to that. I also thought this was neat because for those of you who, like me, try to keep up with all the data updates the last five to ten years, and there's a lot of changes. He does a good job explaining kind of in the last ten years what his career has been and how it's evolved along with the market and then what this new product for him sort of does to try and accomplish the new needs that the market has. And so whether you're really excited about his product or just want to understand more about sort of the last ten years and what's changed in data and tech, I think this is going to be a really interesting lesson. Tarush, welcome.
Tarush Aggarwal [00:01:51]: Jess, thank you so much for having me on the show. Looking forward to being here and hopefully adding some value to your listeners.
Jess Carter [00:01:58]: Absolutely. I think that you have a lot of value to add, so I'm super glad you're here. Okay, so I want to get into, you have this really interesting background of how you became the founder and CEO of 5X, and it sort of evolves out of your technical roles and your understanding of problems around data and a solution to it. Do you mind just taking us right into that?
Tarush Aggarwal [00:02:21]: Yeah, absolutely. Went through a pretty typical engineering background, studied computer engineering, got a job right out of college at Salesforce.com as a software engineer and very quickly realized that software engineering just isn't my cup of tea for many reasons. And somehow found myself being one of the first data engineers, the first data engineer at Salesforce and working on back then, these really interesting problems around, how do you help organizations, like large organizations, actually get value from data, actually build pipelines, sort of structure this model? This is before we had Snowflake and Mixpanel and the Amplitudes of the world. So all of these were very manual problems. And I got to be very narrowly focused, spent my career very largely focused on helping big companies solve these data problems and most recently ran data for WeWork, built a 100-person group, spent a sort of decade doing this.
Tarush Aggarwal [00:03:32]: And a lot of this journey made a lot of sense because the infrastructure layer of data hadn't really been built. We didn't have Snowflakes and we didn't have Fivetrans and there was no DBT and BI tools weren't at this level of majority. So, post-WeWork, I sort of found myself taking a little bit of a sabbatical. WeWork’s IPO has started to fail. I had found myself on a ten day vacation in Bali, and that happened to be day one of COVID and everything in my world is falling apart. Literally, the whole world is falling apart. It was a very interesting time because realizing that the world had changed, the world had changed from what it was ten years ago. We built a lot of tooling and technology over the last ten years to go tackle the data problem.
Tarush Aggarwal [00:04:27]: But the way it was really structured was really backwards. The way data is structured today, it happens to be one of the most fragmented ecosystems and we have 500 vendors and we have 30 different categories. The analogy I'm using is that all of these vendors today sell car parts. And just how crazy this is, is, if you walk into a Honda and they tried to sell you an engine instead of a Honda Civic, you would just leave. But in the data world, this is very acceptable. Like Snowflake happens to be the 100 billion dollar data company. Snowflake is the data warehouse. But what about ingestion and modeling and reporting and reverse ETL and catalog and observability and product analytics and CDP, what about all of that? And the answer of buy this from us and buy something else, go buy ten different sorts of car parts and stitch this together.
Tarush Aggarwal [00:05:23]: This probably worked until last year when there was just a big promise of data, and everyone wanted to be data-driven and there was unlimited money in the economy. But the world has changed and open checks for your data team is just no longer a thing, right? So what 5X really is, is I think it's the beginning of the evolution in data where, hey, how do we give you a custom data platform, which is really built for your industry, your use case, your size, your budget? So take advantage of the sort of multiple options of these vendors inside the data space, but be able to give this to you as an end to end platform.
Jess Carter [00:06:04]: So here's one of my questions from growing up. It was Apple and Microsoft software to me was those two things, you figure it out in Excel because numbers are in Excel or maybe whatever, one way or the other. Now, I've been a Mac user my whole life, since I was a kid. I'm on a Mac right now. I struggle, though, with, it feels like the software and technology market realized they weren't going to break into those. Like they weren't going to be the next Apple or Microsoft. And so then we started doing these slices of a product that can do one piece, a product that can do your warehousing, a product that can automate your ETLing, where you're, you're a product that can help you with your data vault philosophy, if that's your jam. And so I feel like we went from huge solutions that could do everything to little bits where we can carve out our piece in the market.
Jess Carter [00:06:50]: And now it feels like, in your solution, like this return back home. How does that feel when I lay it out that way?
Tarush Aggarwal [00:06:57]: Do you know, I think technology goes through cycles, right? And Apple is a little bit different and Microsoft as an operating system, a little bit different because that's very fundamentally the operating system of your computer. But if you look at like MSN and if you look at Yahoo, those were large platforms, large portals, which did many things. And in general, if you look at what's happened is that we've seen the decline of these large platforms because all of a sudden you had small services which could come and just do one thing much, much better. Let's look at a modern day example, Google Apps or Google Business. It sort of gives you the entire suite, it gives you chat and it gives you video and it gives you email. But we still end up paying Zoom for video and we still end up paying Slack for messaging because we're starting to see that sort of companies want, that consumers want best in breed. I feel that what we've seen is that especially in the data space, which is relatively super nascent compared to a lot of these things, we just went extreme in terms of fragmentation, of like, hey, let's just do something really small. But as I think about what does the landscape look like in the next ten years, I really like this idea of you have a thin application layer on top of multiple disjointed services to bring it together, because both fragmentation and sort of one size fits all, they both have pros and cons.
Tarush Aggarwal [00:08:41]: Right. The problems with one size fits all is that no one wants it. One size fits all in reality, makes sense for no one. And companies want best in breed, but handling multiple best in breed, and especially in a world like data, where everyone's using a land and expand model and interacting with ten different vendors who are each trying to go step on each other's toes, there's just a lot of redundancy, and you spend a lot of your time figuring out the integrations and going and building your car and not enough time sort of going to drive. Building the car works for large companies, which can go deploy armies of data people. But as the world becomes more data driven, that's not your answer for, like, 95% of companies, because for 95% of your companies, building a car is not a competitive advantage.
Jess Carter [00:09:33]: Right. Well, and if I think about the last CIOs, the last ten CIOs I've worked with or CDOs, it's interesting because you're even bringing up, there's flavors, right? There's a flavor of, I just want to manage all my vendors, I'll outsource it all, but I want to have my vendors and trust them and if something goes weird, I'll bring in this other one. I've got redundancy, I've got coverage. If one of them is no good anymore, I'll bring somebody else in. Then you have the CIO that's obsessed with infrastructure structure. I got to support and control my infrastructure.
Jess Carter [00:10:02]: I need to grow it, expand it. I need it to scale better. I want to make a change. It's like they're kind of obsessed with the control they have over the infrastructure. I'm being a bit myopic, but then there's this sort of the data leader, the person who sees your data as an asset, and they care way more about how much all these other things, vendors, infrastructure, bring it to bear than they do about the infrastructure in and of itself or the vendors in and of themselves. They don't want to spend their days talking to a vendor unless it's about how to get their data more effectively, faster, better, stronger. Does that resemble kind of your experience?
Tarush Aggarwal [00:10:41]: Yeah, for sure. There are different components to it. Right? Like, managing vendors is one piece to it, and that's typically very administrative, heavy, especially as you grow size. You involve legal and billing and procurements. So that's one part of it. Right. The other part of it is for your data team. What does this really mean for, hey, I'm switching between six, seven tools on a daily basis.
Tarush Aggarwal [00:11:08]: That just can't be the most productive thing in the world in terms of you just need larger teams to go do this, to basically go do this stuff. Right. And the third piece then comes around the integrations and the cost optimization and all of these different things which didn't really exist. Right. Like CIO was one talking about this in the last few years, but all of a sudden it's become one of those three pillars. I think as we think about the future, as we think about the evolution, what CIOs are really starting to think about is like ROI. And it's not impossible to have ROI building a car, but it just becomes a lot harder. And if you can give someone the right flexibility where you can have a system built in a modular way, go get started off with this tool.
Tarush Aggarwal [00:12:02]: And as it's adding value as the business scales, you can go scale up or for some reason the business isn't scaling or this isn't the product line you can scale down. And when you look at the world inside a sort of modular way and allowing you to actually go focus on the important things with the ROI in perspective, I think that just sort of sets you up in a way to have the ROI conversation very early on, instead of the old approach, which is I'm going to buy a million dollars of infrastructure and I'm going to hire ten people before you have your first use case. But sort of trust me, this is the way to do it.
Jess Carter [00:12:37]: Right! That's the trump card, right? Is whether you're a vendor management, outsourced CIO, or data asset or infrastructure control, which usually happens on highly federated industries, right, where there's just a lot, you got to protect everything. The winning approach, I think, is starting to see your data as an ROI, as an asset, as actually something that can generate revenue. And I think to your point. So I was going to ask you then a question I might have is, do you have a client story? Do you have an example of somebody who's jumped in on 5X and they're starting to really get a sense of the value of the platform and then maybe if they want to take on more, like you just said, that we're kind of seeing that and we're able to understand and appreciate the value of it?
Tarush Aggarwal [00:13:24]: Yeah, absolutely. We happen to really have Fintech as some early adopters. So some of our biggest companies, Fintech, SaaS, and ecommerce, are largely our three verticals. One of our early adopters sort of came to us when they were a five-person data team. They had a warehouse, they had a sort of BI tool, but wasn't really a holistic end to end platform. And we built them back then, a core analytics platform, ingestion, warehousing, modeling, reporting. It's really interesting because sort of this month, they are now a 40-person data team. They have multiple other categories.
Tarush Aggarwal [00:14:09]: They have product analytics and they have reverse ETL, and they have a customer data platform. And they're getting into sort of using LLMs on top of this semantic layer for anyone in the organization to answer questions using conversational BI. And one of the coolest metrics is that at a 40-person data team, they have zero data infrastructure engineers or zero data platform engineers. And for context, at WeWork, when I was there at 100-person data team, we had about 20% of the team, also of 20 data platform engineers. If you think about it, in America, in New York, San Francisco, at about $200,000, which is the average cost of a senior hire, we're spending $4 million a year on building and managing a data platform. We're not doing anything that breakthrough where if someone back then came to us and said, hey, we'll charge you a fraction of that, and here's a managed end to end platform that should have been a no-brainer. So what we get really excited about, as we think about our use cases, our customers typically are about 300-person plus companies.
Tarush Aggarwal [00:15:30]: They're at this point where they found product market fit, or close to finding it. They want to go scale the business, they want to go invest in data, and they might even go take a stab at doing this. But what they very quickly realize is, just like in marketing, making a few posts on Instagram isn't going to make you a marketing company in that same way building a few dashboards inside Tableau isn't making you a sort of data-driven company. And there's a lot more to know. That's one of the sort of success stories which we get very excited about is how do we really do?
Jess Carter [00:16:07]: Like, when they started with you guys, did they already have a warehouse? Was that already in existence? And so how data savvy were they when they started working with you and had the sense that they needed something else?
Tarush Aggarwal [00:16:18]: I think more and more, what we're seeing is more and more companies. We have two or three big different types of customers, right? So what we see is a bunch of companies who might have some of the pieces right. They might have a warehouse, they might have a BI tool, so they've taken a first stab at it and they’re at this point where they realize that there's a lot more to it than just having a warehouse and a BI tool. Right. There's a lot more which goes into this. There's a lot more process. They start having multiple sources of truth. Numbers don't match.
Tarush Aggarwal [00:16:53]: Everything takes super long to update. This sort of business loses trust in the data team. That's very typically sort of pains you see in early stage sort of data teams where they're trying to go build trust in the organization. So we see a lot of those customers, obviously, we see a bunch of newer companies which are looking to build a platform from scratch. But more and more, about a year and a half since we really launched, we're seeing companies who spend sort of millions of dollars or hundreds of thousands to millions of dollars, who are managing ten different vendors and are now realizing that, hey, I don't want to go manage, I want to consolidate all of this. If you look at enterprise software, like a few years ago you had Microsoft and also Oracle, right? So we're seeing more and more our newer deals happen to come from companies who already have these pieces, and they're sort of realizing that they're spending way more money, they're taking way more time. And it's because, number one, are you using the right vendors or this just happened at some point and it hasn't been updated?
Tarush Aggarwal [00:18:04]: Number two is, are you using the vendors in the right way? Have they upsold you on a bunch of stuff you don't need, or was this set up by someone else? And because you have so many of these vendors, you have a lot of duplication, and you're paying for this duplication and largely the time, resources, your data team efficiency in actually going and manage this. So it's either one of these three reasons that sort of companies are starting to come to us and being like, hey, can you manage our entire platform and sort of give this to us as an end-to-end experience like the Databricks of the world, right? How are we recreating Databricks? What Databricks does is end-to-end platform. They have Databricks, ETL and ingestion and Spark and all these things. But again, it goes back to that one-size-fits-all, right? I don't want one size fits all. We're building an end-to-end platform like Databricks. But the difference is we're doing it with the best-in-class components. We're giving you a sort of modular way to go do this.
Jess Carter [00:19:03]: That's so cool. I think it's funny to me, it's a little bit funny to me when you talk about clients or customers who start to realize some of the inefficiency of having multiple vendors, or, hey, do I have the right vendors? It is humorous to me that in data, some of the things we don't measure easily or innately measure is like the number of hours it takes me to manage a vendor and what that should be, or how easy it should be for me to look at the efficiency of my team and understand what that efficiency could be. Or to your point, the ratios of my team and what I could do if I wanted to shift those a bit and maximize some of the team I have. What tools do I need to look at my tools and my people together and look at the business outcomes I want and understand all the ways I can change that. Because if I have all these different vendors, they're just going to tell me exactly why I can't change anything they're doing unless they just take on more. And it's not bad. They're good people who are trying to do a good job in their job. But there's just this sense of the way the game is laid out for all of these people to look at a bunch of data.
Jess Carter [00:20:11]: It's like, just don't look at the data about ourselves or how we're organized.
Tarush Aggarwal [00:20:17]: I find it really interesting. I don't know. I can't go back and put a date on when it became from actually adding value to the business to instead going out for vendor lunches and conferences. It happened at some point in the middle. I just don't know when it happened, but it's become more of that. Like, how big is your team? What tools are you using? Are they the coolest tools? We've actually forgotten that the whole point of this was to actually get an ROI for the business. And all of those, I don't know, whoever wrote those, McKinsey, all of those companies, which data is the new oil and every company should invest in data. It sort of created the right on both sides, from the perspective of data teams as well as from the perspective of executives who want to invest in data.
Tarush Aggarwal [00:21:04]: There were a few years where we were completely blindsided by what's actually happening, and I think we're very excited for what's happening now because people are waking up to this.
Jess Carter [00:21:14]: Well, and they were excited. I agree with you on the blindsided piece. I mean, I remember there was a moment, right, where there was almost this momentum in data and tech, and suddenly you had like FOMO, your fear of missing out. If I haven't touched this one new product that's on the market, I got to have access, I got to have an experience around it. And then you have companies that are buying them because they want to retain their talent, because their talent wants to play with the new tool. This really happens. And it seems so bizarre to me, like there was value, but I do think it's like in the last ten years, there's been this moment, and you're right, I can't pin down quite when, and it's different for everybody, but this sense of it's shifting so fast that I do think a lot of this tech and data industry bubble is trying to keep up with each other, and there's this fear of, I just don't want to miss out, I just don't want to be left behind. Yeah, I think that's real.
Tarush Aggarwal [00:22:11]: And it's not just on the infrastructure. Right. I don't think this is the fault of data platform companies or data vendors. There are two parts of data. One of them is platform, but the other part is people. When analytics engineering became a thing, they were a bunch of people who were like, hey, our job is writing DBT models. That's what we do. And I think we, again, what we ended up doing on the people's side, again, the fear of missing out resulted in building all of these different roles, which necessarily didn't map to adding business value.
Tarush Aggarwal [00:22:49]: An analytics engineer is one small component. I would argue that Maxime wrote this paper called the Rise of the Data Engineer. It began around 2012, 2013, but he wrote this paper in 2017 that was very relevant because at that point, we didn't have pipelines, we didn't have data modeling to meet a certain quality. So the data engineer became really a sort of very critical component needed in order for companies to extract value from data. That's what we were doing from 2011 to 2017.
Jess Carter [00:23:22]: That's right.
Tarush Aggarwal [00:23:23]: And we created a bunch of titles after that, but they were just very sequential. Hey, the data engineer does this and the next person does this and the next person does this. I would argue where we are actually going now is the rise of the data generalist where, hey, we have all of the right tooling in place. I want to have a sort of micro data team where a small data team can actually go do all of the different pieces and actually look at this holistically without needing more dedicated people to actually go produce the ROI, right? Platforms like 5X. And I'm not saying like we'll be the only end-to-end data platforms, but I think this is going to begin a trend. It's a new category. It's sort of going to be called fully managed. You should be able to go manage your infrastructure holistically, end to end, depending on your budget, your industry, the capabilities you need, and give you a platform.
Tarush Aggarwal [00:24:16]: And now an actual data generalist should be able to go ingest your data, build your models, build out your sort of BI dashboards, be able to push this data back into other sources, go figure out what your engagement is. So I'm really looking forward to this sort of rise the data generalist.
Jess Carter [00:24:35]: I like it. I think it's cool because I think we should take on the FOMO. I think we should handle this right now. So if I ask you, I'm going to answer first, but if I ask you what are some ways that you would encourage anyone in data where they can avoid ever being left behind? And I think my answer would be, I've seen people in all of my client situations where someone is obsessed with a new philosophy. They want to talk about data vault, or they want to talk about agile leadership, or how we manage a safe practitioner program, all of that can be good. But if it becomes this thing where you're so obsessed with the how we're working or the how in the principles of it, that you want to follow the new rules and try the new thing, usually you've lost the why. And I'll watch people lose that connection to the business and the return on investment. And if we actually made a meaningful change or outcome, or if we just wanted structure in an industry that is notorious for change.
Jess Carter [00:25:43]: And I think some people are in data and tech and they just want structure. Like just let me have the framework and then get out of my face and I'm like, I think you can get left behind if you do that. I think you can be really good at something, but you'll miss the business value and you'll end up wondering what happened to you. And the reality is we lost touch with the why we're here in the first place. That would be mine. What do you think?
Tarush Aggarwal [00:26:08]: No, I think it's a super valid question, right? I think change is going to happen anyway, right? I remember again a few years ago when sort of Fivetran first came out, the normal response by data teams was, why would I use Fivetran? I've already built my own pipelines? Just because you've built your own pipelines doesn't mean it's the right thing to do. And what Fivetran allow you to do is build automation on top of it by sort of standardizing how ingestion works, we can now start to build layers on top of this. I think that's the same thing which we're seeing with something like 5X, right? Companies like, oh, but I've already built and integrated my platform. Just because you've done it doesn't mean it's the right thing to do. You shouldn't be managing and maintaining this moving forward.
Tarush Aggarwal [00:26:51]: So I think change happens anyway. And people who are very gifted in building pipelines, I'm not saying there are no pipeline jobs anymore, but in general, majority of those have gone and abstraction moves upstream. So in general, the analytics engineer was the next evolution on top of your initial data pipeline engineer. And to some extent it's becoming data sciency stuff now. So it's happening whether or not you are aware of it. So I think now that you have the awareness, I think in general, seeing that if companies are going to get focused more on ROI and if companies are going to start to care about how much they spend, then you have to agree with me that one of the best things you can do is actually how do you build a sort of diverse general skill set in the data space today? Because that is going to keep you relevant in terms of being able to add end to end value. I think my answer is, I think in this generalist to be broader. And it's funny because I don't think generalists really, I think there'll always be value for, obviously specialists and organizations which are niche or very large, you will have that.
Tarush Aggarwal [00:28:15]: But I think when we look at sort of data generally and we speak about it right, we speak about it from the perspective of, when I speak about data generally, I speak about it from the context of a 300 to a 2000 person company or a ten to 50 person data team for companies like this. I think your sort of generalist in general is just a much better route to code today than it was two years ago.
Jess Carter [00:28:45]: Well, and you're also currently combating what I think is one of, I think you said this, but one of the, maybe the two things that people have FOMO about right now to me is sometime in the last couple of years, suddenly everyone needs a data scientist when the market couldn't even agree on what that really is. And then I think there's also AI. I think those are the two things where it's like, if I'm not using them, I'm behind. And it's like, it's easy, everybody. I'm not saying that those are bad things. Those are great things. We have data scientists, they're incredible. But I think we have to be careful about, again, why do we need them? What are we talking about? And I've had data scientists who also know how to handle some of the piping and actually understand how to cleanse their data.
Jess Carter [00:29:24]: So there's this broad variety of, like, what do we mean when we even say that stuff? And what is the best way to approach if my business actually would benefit from it? And if so, how?
Tarush Aggarwal [00:29:34]: Yeah, that is a huge rarity, right? Data scientist who knows how to build their own pipelines. That's how it should be. Because if they're depending on another team to do it, they might not be sort of very relevant a few months from now. But I think when it comes to data science, I think it's really interesting because I think 95% of companies are going to end up using a data science product to core get insights. And only 5% of companies need to be a data science company. We speak to ecommerce companies who are not small, like fairly large. They might be like 10 million plus in terms of revenue, or 50 million plus, even for larger businesses, unless you're massive enterprise scale I still don't think there's ROI in building out some of these models yourself.
Tarush Aggarwal [00:30:32]: At some point, a lot of this stuff gets commoditized and a platform and a vendor will just do it much better for you. Data science was such a, I think data science started becoming a hype. Initially, data science was so hyped, our infrastructure wasn't ready to go do that. We didn't have clean, reliable model data in a way which made sense for data scientists, but the sort of title sounded cool. And now that actually we are ready for data science, what's happened is that the application space has evolved so much that your data science can't keep up with actually an application which does data science for you on top of your structured data. So we missed it on both sides. We were too ready for it, and now that it's ready, it's no longer relevant.
Jess Carter [00:31:22]: I think it's funny. I appreciate that, because I do think the other thing I'm noticing is middleware. Like any mid market software company is trying to integrate AI and insights anyway. And so it's like if you're buying a product, largely, it has its own insight engine, it's trying to generate for you. But to your point, not custom, not modularized. And so I think it's cool that you've kind of taken on that. That to me, seems like a unique niche for you in 5X is that it's like, hey, we're going to do this in a way that scales nicely, but also we can do this in a modular way.
Tarush Aggarwal [00:31:57]: We aren't even talking about data science, to be honest now, right? They're like 15 different categories just in the data analytics space, ingestion, storage, modeling, reporting. So I think data science is something which we'll think about as to once you have built yourself a data platform, how do we now go plug in the pieces which get into the insights recommendations? We're doing our first ones now around sort of LLMs on top of the semantic layer. So if you have a well-defined data model, you'll just be able to go plug in a sort of LLM on top of that. And in Slack you're like, hey, how's last month's numbers and how did they compare to the month before that? And which of my channels went up and down? And is this actually significant? And what would you think I should do? Like answering that question in Slack is, I think, a much, much more sort of evolved experience than opening up Tableau and trying to go answer those questions.
Jess Carter [00:32:57]: This is a journey I'm on right now, right? So we built a warehouse, and I run our resource management team at our company. So when we've got projects for companies, I'm constantly looking at the staffing, like, do we have 200 consultants? One of the things we just realized when we kind of made the data more accessible to me is I can now have a view I didn't have of, hey, in 30 days, 60 days and 90 days, what percent of our staff is really staffed? And it turns out there's some trends there of like, a certain percent usually means it's okay, and a certain percent might mean, hey, we're in a bad way. But it's a crazy process to go through to take my own medicine here and not just be consulting, but now have access to my own data to drive insights and be like, man, I wish now that I have the Tableau dashboard or whatever it is, Power BI where I can see the data. Now I have this next maturity of how do I generate insights? How do I better understand how it's moving? How do I generate and attribute meaning to it to appreciate when I need to change my behavior for a better outcome? And so I feel like I don't know. Part of me gets frustrated by the journey, that it feels like it is one bite at a time of the elephant, of like, I see it all. I've coached and consulted, but taking my own medicine, I have to take it one pill at a time.
Tarush Aggarwal [00:34:15]: Yeah, for sure. And I think I've gone through that in the last 18 months. Right. I've spent my career very much been in the data team seat. And being a CEO now, it's a very different hat because data is important, but it's just a very different view as what I had when I was running data for a company versus going and running business and then using data as that lever. And it's a big learning experience for myself because so many things which I thought were important in the data world, when you look at it from a business lens, just aren't important. It's just noise. And it's a difficult thing to share because unless you have that experience as a data, being a data practitioner, you can be enthusiastic about sort of so many things.
Tarush Aggarwal [00:35:09]: And I don't think it's an easy muscle to actually go to end up which one of those things are actually important for the business and which one are important because that's your job.
Jess Carter [00:35:20]: That is the summary of exactly where I find myself today at work is this sense of man, we've been preaching. And now I feel like I'm taking my own medicine, and I'm like, oh, this is trickier. And you're right. There are things I thought were important, and I'm now like, oh, actually, this is important way over here. And I never would have thought about it. So I love the distinction of running a data team versus running a business with data. We've done both, and they are two very different animals. Well, hey, I know we're running up on time.
Jess Carter [00:35:57]: Is there anything we haven't talked about yet that we definitely, definitely should?
Tarush Aggarwal [00:36:01]: I'm glad we had a small pit in the end towards data science, because that's a very popular topic.
Jess Carter [00:36:08]: Good.
Tarush Aggarwal [00:36:08]: No, I think, yeah, this was a really fun conversation.
Jess Carter [00:36:12]: Good. Okay. If people want to follow you, where can they kind of reach out and keep in touch with you?
Tarush Aggarwal [00:36:19]: That's a great question. LinkedIn and Twitter are probably the easiest. My name is Tarush Aggarwal. Tarush is one of the rarest names, and Aggarwal is one of the most common names. Net should be, I don't know, easy to find. And on Twitter, I'm just Tarush, being a super rare name. I was able to get my first name, which I should probably use more.
Jess Carter [00:36:46]: That's a big deal.
Tarush Aggarwal [00:36:48]: On 5X, it's like, literally the shortest domain name. It's 5X dot CO.
Jess Carter [00:36:55]: Awesome.
Tarush Aggarwal [00:36:56]: Super easy to find.
Jess Carter [00:36:57]: Thank you for listening. I'm your host, Jess Carter. Please don't forget to follow us on Data Driven Leadership wherever you get your podcasts. And rate, rate and review, giving us some feedback on how these data topics are transforming your business. We can't wait for you to join us on the next episode.
Insights delivered to your inbox