In a previous article, we discussed how defining artificial intelligence (AI) and machine learning (ML) ethics and establishing governance best practices can help you achieve short-term gains and long-term successes with your AI initiatives. Given the direct link between ethics and regulation, there are also key questions that legal teams should be asking to assess risk and inform decision making during the build phase of AI/ML endeavors and beyond.
With existing regulations already being applied to AI and new regulations on the way, organizations need to create a solid foundation for navigating compliance and ensuring that AI/ML models adhere to regulatory requirements, as well as internal governance for ethical AI/ML
These 10 questions were adapted from letters sent by Senators Cory Booker and Ron Wyden to the heads of major healthcare companies in December of 2019. The letters were in response to research that indicated a widely used algorithm was discriminatory. These types of questions reflect the increased regulatory oversight of AI/ML in the short- to medium-term.
1. How many AI/ML models, including third-party models or those that serve as inputs into other models, does your company deploy?
Depending on your industry, you might be tempted to separate formal quantitative models (think SR 11-7 for the financial sector) from the rest. Think again! A regulator can identify a model in a much broader sense. The safer path is to examine which models make decisions autonomously and directly or indirectly affect an individual, whether an internal or external stakeholder.
2. What types of outputs or recommendations is each model making, and where is documentation about these models stored?
You will need a pre-determined criteria in place regarding the impact and severity of the decisions that the model makes (your operational risk management staff can be helpful here), and the associated dangers with those decisions. Regulators will want to see that you have thought this through and established quantified and qualified mitigation steps to reduce both liability and harm to an individual.
You can store the location, input, output, and even the types of impact of the models in a variety of places. You can choose an application portfolio tool or a data catalog/ governance tool. You can even create a workflow in a collaboration platform. The goal is to make it easy for anyone looking at the output of AI/ML to trace back to the purpose, outcome expectations, validation, and testing of the model (if applicable), and the business process affected.
3. How many people or organizations does each model potentially impact?
Volume counts when it comes to the effect of a harmful outcome as a result of poor outputs from the models. Your best bet is to contain stakeholder impact until your models have been properly tested and validated, and proper AI governance has been applied. Then you can extend the outcomes to a wider group of people, both internally and externally.
4. How are your organization’s models audited for security or privacy vulnerabilities?
Take a shortcut and leverage what is already in place. Use the National Institute of Standards and Technology (NIST) AI Playbook and the NIST Security Software Development Framework (SSDF) for proper development of any tool, and review the Federal Blueprint and associated executive order.
These four guiding sources should cover all questions that could be posed to your organization in terms of how well you are governing your ML/AI from creation to final deployment, and decisions being made as a result of the outputs. You should be well-prepared for any audit or security/privacy inquiries regarding AI.
5. Given the substantial harm that can be caused by incidents such as attacks or failures of AI/ML models, does your company have AI/ML incident response plans in place?
Save yourself a lot of trouble by obtaining a robust AI incident response template and having it ready for any crisis. Your plan should cover an end-to-end response plan that includes you, your business partners, and your vendors. This type of plan can guide everyone to the fast track towards compliance and will help you with contractual clauses and vendor dialogues.
6. Does your company audit models for AI/ML-related liabilities before a model is deployed? Are different audit processes applied for different types of models?
Regulators are looking to determine whether you have thought this through and have tiers of effort and time placed into the activities related to your internal testing/audits. This does not take a lot of time and will help bring the focus to what matters most based on the scope and effect of the automated decision-making process.
7. Does your company monitor models for AI/ML-related liabilities during deployment? Are different audit processes applied for different types of models?
The answer here is the same as question 6. Internal testing and audit activities should be closely monitored, prioritizing models with the greatest scope and effect.
8. Have you quantified sociological bias in your company’s AI/ML training data and model predictions? Is your company aware of how each model affects different demographic customer segments?
This is where the Federal Blueprint really helps. Using this guidance as controls in your internal framework, you can easily segment demographics that would be negatively affected by mistakes or issues related to the model you are implementing. Use the Blueprint as a reference for targeting segments of your customer base and, at the same time, include checkpoints of review to minimize dangers.
9. Several organizations have published detailed standards or best practices for “trustworthy AI.” Does your company utilize any of these resources when implementing AI/ML? If so, which ones?
This is still a fairly new space with regards to end-to-end governance, so stick with the most vetted and trusted sources. You can never go wrong with the standards coming from NIST, the Office of the Comptroller of the Currency (OCC)/ Federal Financial Institutions Examination Council (FFIEC), and the Federal Trade Commission (FTC). If you wish to be even more proactive, consider reviewing what AI auditors will want to see. The ForHumanity.Centre website is a solid resource for this.
10. Have any independent third parties or other external experts (legal, security, or others) been involved in your company’s procedures to address the known liabilities of AI/ML?
Regulators want to see that you are showing that you care about this by investing time, money, and people into a task that will have lasting effects. This is not a necessity, but it brings a degree of comfort to those evaluating you if you have brought in experts to assist you along the way.
A Proactive Approach to Ethical AI and Compliance
Companies in heavily regulated industries like financial services or healthcare are no strangers to regulation. Many have taken proactive steps to ensure compliance as AI/ML solutions are deployed. However, AI will impact every industry. All companies should consider the ethical and regulatory implications of their AI/ML initiatives and ask these questions. Reach out to Resultant if you’d like to discuss how you can successfully navigate AI while minimizing risk.
Share:
About the Author
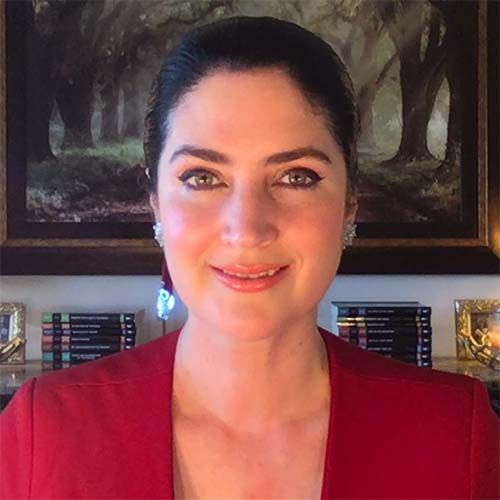
Paola Saibene
Principal Consultant @ Resultant